Data-driven modeling
Data-driven COVID-19 modeling
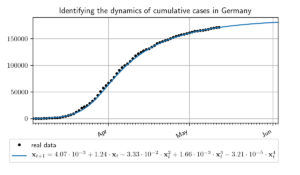
The work around this topic is aimed at exploring the usage of a system identification tool called “sparse identification of nonlinear dynamics” for COVID-related data. So far, three directions were considered: (1) assessing the predictive power of models identified from the evolution of cumulative cases in a single country compared to classic time-series forecasting tools. (2) Including additional information about health, sanitation and measures in each country to study the influence of these factors on the evolution of the pandemic. (3) Identifying the dynamics from the evolution of susceptible, infected and recovered quantities in different countries and comparing them to classical SIR models.
A more complete review of this activity can be found here:
Cyprien Neverov, Enrique Zuazua